Data analysis is at the heart of decision-making in businesses today. With the explosion of data, traditional methods are struggling to keep up. Enter AI tools, which promise to revolutionize data analysis by enhancing speed, accuracy, and insights. But how exactly do these AI tools improve data analysis processes? Are they the unseen revolution transforming industries, or just hype in the tech world? Let’s explore how AI tools enhance data analysis, diving into their features, benefits, and real-world applications.
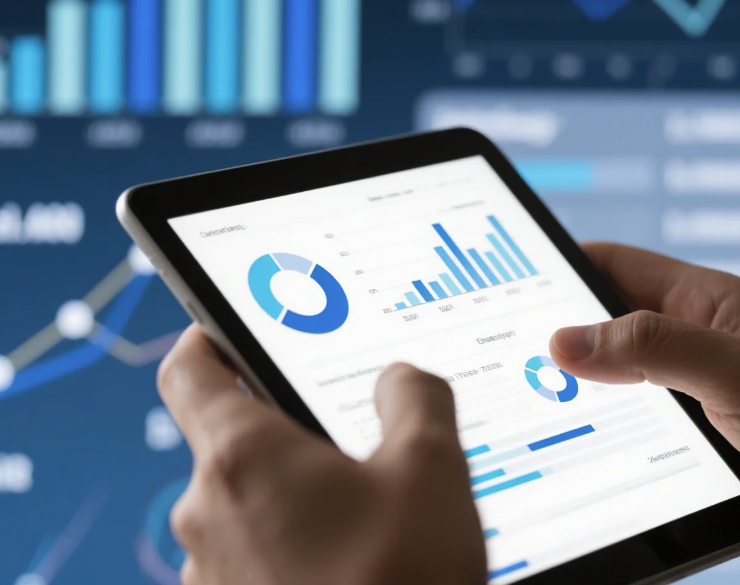
The Traditional Challenges in Data Analysis
Traditional data analysis involves manual data collection, processing, and interpretation. These tasks can be time-consuming and prone to human error, especially when dealing with large datasets. Analysts face challenges such as data silos, complex data structures, and the need for real-time insights.
Traditional vs. AI-Driven Approaches
While traditional data analysis relies heavily on human expertise and manual effort, AI-driven approaches offer automation, advanced algorithms, and enhanced data processing capabilities.
How Do AI Tools Improve Data Analysis Processes?
AI tools in data analysis come equipped with features that significantly enhance the efficiency and effectiveness of data handling. Here’s a closer look at some key features and the tools that offer them.
1. Automated Data Collection and Processing
AI tools can automate the collection and processing of data, reducing the time and effort required.
Example Tool: Alteryx
Alteryx uses AI to automate data preparation, blending, and analysis.Features: Automated data cleaning, integration of multiple data sources, and intuitive data workflows.
Benefits: Saves time on data preparation, enhances data accuracy, and supports large-scale data handling.
Why It Stands Out: Its user-friendly interface and powerful automation features make it ideal for complex data environments.
2. Advanced Predictive Analytics
AI tools provide advanced predictive analytics, offering deeper insights and foresight into trends.
Example Tool: IBM Watson Analytics
IBM Watson Analytics uses AI to deliver predictive analytics and data visualization.Features: Predictive modeling, natural language processing, and interactive data visualizations.
Benefits: Enhances decision-making, uncovers hidden patterns, and supports strategic planning.
Why It Stands Out: Its integration with IBM’s cloud services provides scalable solutions for businesses of all sizes.
3. Real-Time Data Analysis
AI tools enable real-time data analysis, allowing businesses to act quickly on insights.
Example Tool: Tableau with AI Features
Tableau’s AI capabilities support real-time data analysis and visualization.Features: Real-time data streaming, dynamic dashboards, and AI-driven insights.
Benefits: Improves responsiveness, enhances operational efficiency, and supports agile decision-making.
Why It Stands Out: Its ability to visualize data in real-time makes it a powerful tool for dynamic business environments.
4. Enhanced Data Security
AI tools improve data security, protecting sensitive information and ensuring compliance.
Example Tool: Splunk with AI Features
Splunk uses AI to enhance data security and threat detection.Features: Automated threat detection, anomaly detection, and security analytics.
Benefits: Protects sensitive data, improves threat response, and supports regulatory compliance.
Why It Stands Out: Its focus on security makes it an essential tool for businesses handling sensitive data.
5. Improved Data Visualization
AI tools enhance data visualization, making complex data easier to understand and communicate.
Example Tool: Qlik Sense with AI Features
Qlik Sense uses AI to create interactive and intuitive data visualizations.Features: AI-driven data visualization, drag-and-drop interfaces, and storytelling capabilities.
Benefits: Improves data comprehension, enhances communication, and supports data-driven storytelling.
Why It Stands Out: Its intuitive visualization features make it accessible to users without technical expertise.
How to Implement AI Tools in Your Data Analysis Strategy
Integrating AI tools into your data analysis strategy requires careful planning and execution. Here are some steps to guide you.
Step-by-Step Implementation Process
Identify Your Data Needs: Determine what you want to achieve with AI tools, whether it’s automating data processing, enhancing predictive analytics, or improving data visualization.
Select the Right Tool: Evaluate different AI tools based on their features, compatibility with your existing systems, and ease of use. Consider conducting a trial to assess their effectiveness.
Integrate with Existing Processes: Ensure that the AI tools you choose can integrate seamlessly with your current data workflows. This may involve working with IT professionals or consultants to facilitate integration.
Train Your Team: Provide training to your data analysis team to ensure they understand how to use the AI tools effectively. This will maximize the benefits of the technology and improve your overall data strategy.
Continuously Monitor and Adapt: Data analysis is an ongoing process. Regularly review and update your AI tools and strategies to keep up with evolving industry trends and technological advancements.
Potential Challenges and Solutions
Data Privacy: Ensure the AI tool complies with data protection regulations and has robust security measures in place to protect sensitive information.
Change Management: Introducing AI tools may require changes in data processes. Communicate the benefits and provide support to facilitate a smooth transition.
The Future of AI in Data Analysis
As AI technology continues to evolve, its role in data analysis is likely to expand. Future developments may include more advanced analytics capabilities, enhanced real-time processing, and deeper integration with other business technologies.
Emerging Trends
AI-Driven Predictive Analytics: Future AI tools will offer more sophisticated predictive analytics, allowing for highly accurate forecasts and insights.
Integration with Internet of Things (IoT): AI tools will increasingly integrate with IoT technologies, providing real-time data from connected devices and enhancing operational efficiency.
Conclusion: Embrace the Future of Data Analysis
AI tools are not just hype; they represent a transformative shift in how data analysis is conducted. By embracing these technologies, businesses can enhance efficiency, accuracy, and insights. Now is the time to explore and implement AI tools in your data analysis strategy.